LiDAR-based soybean crop segmentation for autonomous navigation
The technological advances in the last few decades have greatly changed agricultural operations. In order to became safer, more profitable, efficient, and sustainable, modern farms have adopted the use of sophisticated technologies, such as robots, sensors, aerial images, and GNSS (Global Navigation Satellite System). These technologies not only increase the crop productivity, but also reduce the wide use of water, fertilisers, and pesticides. Due to this, they reduce costs and negative environmental impacts, such as the contamination of water sources. In special, the use of mobile robots has allowed more reliable monitoring and management of natural resources.
However, the application of robotics in agriculture is still challenging and researchers are seeking to develop smarter autonomous vehicles that can safely operate in semi-structured or unstructured dynamic environments, where humans, animals, and agricultural machinery may be present. The most common sensor types used to enable autonomous navigation are cameras, LiDAR (Light Detection and Ranging), GNSS, inertial sensors, and encoders. Real Time Kinematics GNSS have allowed the autonomous operation of many machinery due to their highly accurate positional information when a clean view of satellites is possible. Nevertheless, such systems cannot handle dynamic obstacles (animals, humans, stubble, or machines) positioned along the robot trajectory. Moreover, their performance may degrade due to uncontrollable sources that affect the satellites signals. To guarantee safety in scenarios where GNNS may fail, cameras and/or LiDAR can be used.
Although vision systems are commonly used in mobile robot navigation, they have some disadvantages in outdoor environments: the main one is the presence of high variation of lighting conditions. Other sensors (e.g.: LiDAR, ultrasound, and infrared) are used to measure absolute distances in agricultural environments. Among them, LiDAR sensors recently have greatly benefited from cost reductions while maintaining a fast, high range, and millimetre-level measurement. In this paper, we describe the deployment of a perception system based on two 2D LiDAR sensors for the navigation of an agricultural robot in soybean crops. The proposed system takes advantage of the robot’s movement, which is tracked by odometry, to create a 3D point cloud by concatenating consecutive 2D LiDAR readings. In order to determine the position of each soybean row, the point cloud goes through a mask to highlight vertical entities and through Gaussian functions to enhance the known/expected row position. Subsequently, the sum of all points for each cell in a plane parallel to ground generates a grid map. A threshold filter is applied to suppress irrelevant information. Finally, a histogram filter determines the position of each row. Aiming to verify the behaviour of the proposed system, experiments were carried out with a 4WSD (four wheel steering and drive) robot in a soybean crop. The robot achieved 22 of 28 runs made, where the length of each run was 32m. Although the robot could not finish 6 runs, we detected that all failures were caused by control system and some mechanical restrictions. Hence, we concluded that the proposed perception system is a promising tool to detect the position soybean crop rows.
Speaker
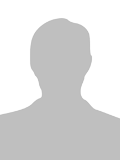