Estimating greensnap yield damage with canopy reflectance: a case study
Grain yield reduction caused by storm-induced plant breakage (green snap) occurs often in corn fields. With climate change and an increasing frequency in the occurrence of extreme weather events, it is essential to develop methods that can accurately estimate green snap damage, so growers can be properly compensated by insurance companies for yield loss. Because plant breakage also affects crop canopy reflectance, this case study aimed to characterize the changes in crop canopy reflectance caused by green snap, and to evaluate the utility of crop canopy reflectance to estimate green snap yield loss.
The study was conducted in Minnesota, USA at the same site where a nitrogen rate study had been established in the spring. In July of 2019, a windstorm hit the study area when plants were at VT stage, snapping 0–70% of the corn stalks just above the ear leaf at the different plots. Damaged and undamaged plants were harvested separately from a total of 125 plots (n = 125). Achievable yield was estimated by extrapolating the yield of the undamaged plants to the entire plot area, and yield loss was determined as the difference between the achievable yield and the observed plot yield. Percent yield loss was given by the ratio of yield loss and achievable yield. Hyperspectral imagery was collected at 4 cm spatial resolution with a line-scanning camera (Resonon Pika IIg VNIR) between 400 and 900 nm at 2.1 nm resolution 12 days before and 2 days after the storm. Image processing included radiometric correction, georectification, transformation to reflectance (empirical line method), smoothing of the spectral domain (Savitzky-Golay procedure), image cropping of the middle two rows from each plot (1.5 x 6.0 m area), and extraction of the average reflectance value for each band within each cropped image. Feature selection was performed with Lasso Regularization. Prediction models with elastic-net, partial least squares, and multiple linear regression were evaluated using the lasso-selected features. Cross-validation was implemented in the training set (n=86) for model training and hyperparameter tuning, and a test set (n=32) was held out to evaluate the final prediction models.
The plots with the most vigorous corn plants had the greatest green snap incidence. Overall, every unit increase in percent plant breakage was associated with a 0.54% increase in yield loss (R2=0.79, RMSE=3.9%, p < 0.01). Crop canopy reflectance in the visible and red-edge spectra were affected the most by the green snap damage, indicating greater soil exposure with plant breakage. A total of 17 features were selected by the lasso regularization procedure. Reflectance in the 501, 725, 680, 505, and 848 nm wavebands were the most informative to predict green snap yield loss, with feature importance of 19, 13, 12, 12, and 12% of the total, respectively. Using the lasso-selected features, percent grain yield loss could be predicted with a RMSE of 5.7 and 6.0% using elastic-net and partial least squares regression, respectively. Multiple linear regression performed slightly worse, with a RMSE of 6.2% and a tendency to overestimate green snap yield loss.
The low out-of-sample RMSE values obtained in this study indicate that there is potential to predict grain yield loss caused by green snap damage using crop canopy reflectance. While this case study provided a wide range of green snap yield loss values, a larger sample size may be necessary to develop robust prediction models that can be implemented in commercial fields. Future analysis on this dataset can be implemented to simulate the effect of pixel size and spectral resolution on the accuracy of predictions.
Speaker
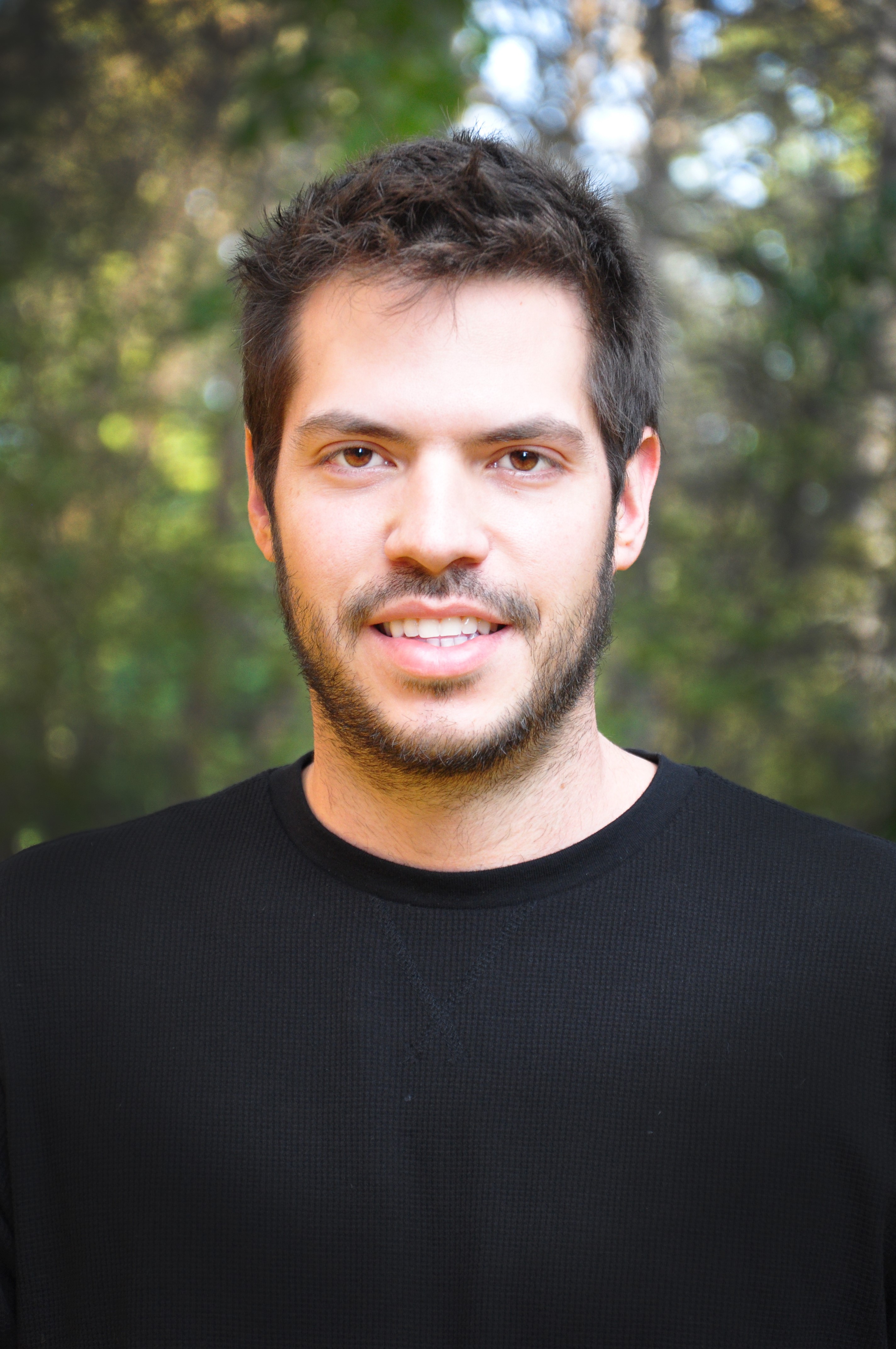