Session Details

Date: Wed Dec 9, 2020
Time: 8:35 AM - 10:15 AM
Moderator: N/A
Africa needs its own and unique Green Revolution now, but it can also take advantage of many lessons learned all over the world. Precision farming is much more than the application of advanced, expensive technologies for managing soils and crops on a commercial scale. In the African context, it is all about better agronomy at any scale, including small and medium-size farms. Many ingredients for that exist already, whereas others still need to find their way into scalable business solutions for this kind of small-scale precision farming. Besides managing soils, inputs and other critical cropping decisions through tailored knowledge and products, suitable mechanization solutions and business models for them will be required to overcome the current lack of energy in the field.
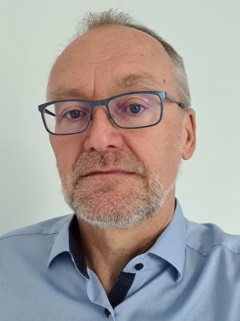
During the last decades, efforts have been made to increase the yield and the quality of major fruits and vegetables but still, farmers mainly those in West African countries are struggling to close the yield gap. Precision agriculture has been reported in most developed countries as a set of tools integrating information and technologies for efficient crop production. Over the decades, scholars have been skeptical about the development and implementation of precision agriculture in West Africa mainly because of the type of agriculture which is smallholders-driven. The present paper aims at grabbing existing precision agriculture technologies in the West Africa to adapt and scale them up in the fruit and vegetable sector. A systematic literature review approach used. Out of the 353 papers pre-selected, 71 scientific papers were selected considering the experimental site, the period when the research has been conducted and the adaptability of the technology to fruit and vegetable production. It came out that there is a lack of research on precision agriculture related to fruits and vegetable crops. The existing technologies have been widely documented for field and cash crops; some technologies were region-specific. Technologies such as seed priming and seed treatment (13% of selected papers) and conservation agriculture (12% of selected papers) are more specific to the semi-arid West Africa where as site-specific fertilizer management (25% of selected papers) is mostly present in humid West Africa. However, low-cost mechanization (6% of selected papers), fertilizer micro-dosing (21% of selected papers) and precision water management (23% of selected papers) are used throughout the West African region. A multi-steps model is proposed for a wide adoption of these technologies among fruits and vegetables farmers. New research initiatives need to be undertaken to identify other low-cost technologies such as handheld sensors which could be used as a decision support tools by smallholders’ farmers.
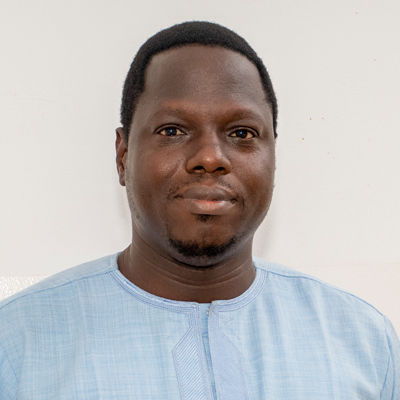
The majority of African smallholder farmers do not have access to soil analytical services. The main reasons are relatively high costs of wet chemical services and difficult logistics. As a result they have to rely on blanket fertilizer recommendations. This often causes poor soil management due to very heterogeneous soil conditions. As a result, the return on investment from blanket fertilizer recommendations is low and fertilizer acceptance is not growing among smallholder farmers. Soil spectral services give promise to overcome these constraints. Soil spectral services are affordable and easy to operate as analytical services. In addition they can be decentralized and run e.g. as mobile lab moving between farmer´s groups. As a result the big mass of yet unserved smallholder farmers could get access to advisory services, which are based on soil analysis. However, calibrating spectral services is an art that needs huge, representative data bases of spectra and high-quality wetchem values. In addition, highly skilled staff are needed to optimize and update models using state of the art machine learning models to serve bigger regions over time with the benefits of spectral services. The aim of this study is to compare for a pan-African mid-infrared spectral database lightGBM regression modelling with the deep learning modelling approach. This was done as an example for three soil properties - pH value and contents of phosphorus and sand. In order to test for modelling the importance of sample number, a varying number of samples in calibration and hold-out validation were chosen. The number of calibration/validation samples for pH value were 51,390/9,068, phosphorus content 47,566/8,393 and sand content 7,052/1,245, respectively. Root mean squared error (RMSE), ratio of standard deviation of reference values to RMSE and r2 values for deep learning on the hold-out validation sets were for pH 0.28, 3.5 and 0.92, for phosphorus content 56 mg/kg, 1.5 and 0.58 and for sand content 7.6%, 3 and 0.89, respectively. RMSE values compared to ligthGBM regression were reduced for the 3 soil properties by 28%, 19% and 14%, respectively. From an advisory perspective very low nutrient concentrations are important as plant response to fertilizer application is highest there. Deep learning could lower for phosphorus content the RMSE calculated on the lowest 25% quartile range (0.13-5.4 mg/kg) by 44%. Moving to deep learning as a modelling tool for soil spectral libraries is a very promising step. The advantage of model performance over current machine learning approaches increases once the spectral libraries pass a few 10,000 samples. Another big advantage from an agronomic perspective is that deep learning provides the biggest relative improvement especially at lower nutrient ranges. The combination of a pan-African soil spectral library with deep learning modelling offers great potential to serve as input for advisory services of the mass of yet unserved smallholder farmers.
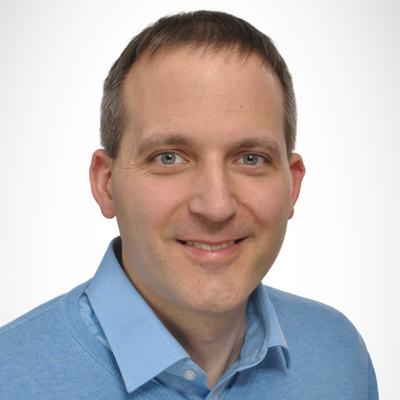
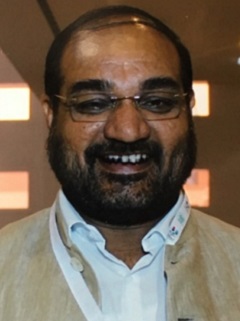
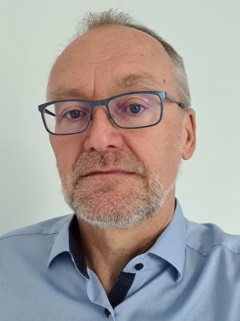
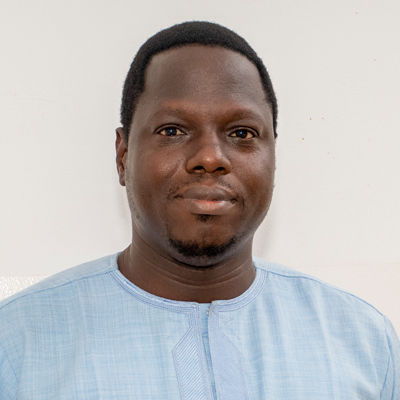
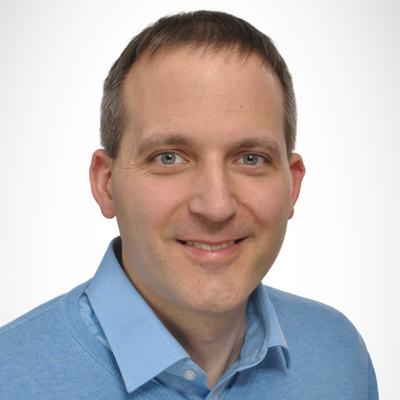
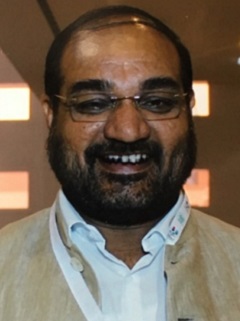